The Ultimate Guide to Data Labelling Tools
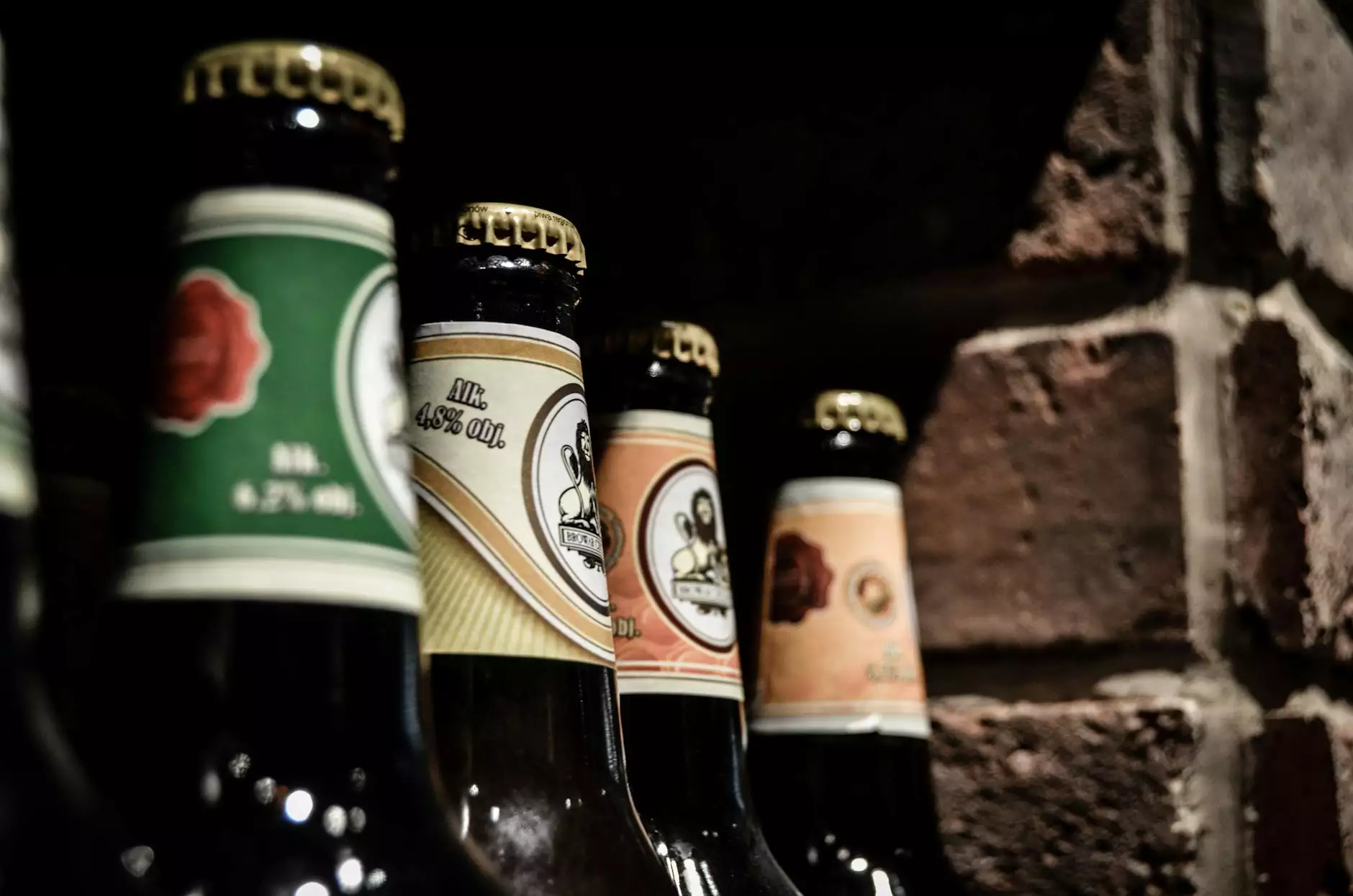
The rise of artificial intelligence (AI) and machine learning (ML) has considerably transformed various industries. Central to the success of these technologies is the data labelling tool, a crucial component that enables organizations to prepare their data for training effective AI models. In this comprehensive guide, we delve into the significance, functionalities, and advantages of data labelling tools, with a special focus on KeyLabs.ai, a leader in the Data Annotation Tool and Data Annotation Platform categories.
What is a Data Labelling Tool?
A data labelling tool is a software application designed to assist in the process of annotating data, which involves the tagging or labeling of data frames to prepare them for training machine learning models. This process is essential for supervised learning, where the model learns from labeled examples in order to make predictions on unseen data.
Why is Data Labelling Important?
Without properly labelled data, AI models can quickly learn incorrect patterns or fail to recognize relevant features, leading to poor performance. Here are several reasons why data labelling is essential:
- Accuracy: High-quality labelled data directly improves the accuracy of AI models.
- Efficiency: Annotated datasets save time during the model training phase.
- Scalability: Automated labelling tools can handle vast amounts of data swiftly.
- Customizability: Businesses can tailor labels specific to their needs and requirements, ensuring relevance.
Features of Effective Data Labelling Tools
When choosing a data labelling tool, certain features make a tool stand out. KeyLabs.ai offers a robust suite of functionalities designed to streamline the data labelling process. Key features to look for include:
- User-friendly Interface: An intuitive interface allows teams to annotate data without extensive training.
- Multiple Annotation Types: Support for a range of data formats, including text, images, audio, and video.
- Collaboration Tools: Features that facilitate teamwork, such as shared projects and commenting options.
- Quality Control Mechanisms: Techniques to ensure the quality and accuracy of labelled data.
- Integration Capabilities: Compatibility with existing data management systems and workflows.
The Benefits of Using Data Labelling Tools
Adopting a data labelling tool presents numerous advantages that can significantly impact a business's operational efficiency and decision-making:
1. Increased Productivity
Automating the data labelling process can drastically boost productivity. With manual labelling often being time-consuming, tools like those offered by KeyLabs.ai enable rapid annotation, allowing teams to focus on other critical tasks.
2. Enhanced Data Quality
Many data labelling tools incorporate advanced algorithms and machine learning techniques to ensure high-quality annotations. They minimize human error and improve consistency across large datasets.
3. Cost Efficiency
Using data labelling tools can lead to significant cost reductions. Businesses can save on the costs associated with hiring extensive annotation teams while still improving output quality through automation.
Types of Data Labelling Techniques
Data labelling techniques vary based on the type of data and the complexity of the task. Here are some commonplace data labelling techniques supported by data labelling tools:
1. Image Annotation
Image annotation involves tagging images with bounding boxes, polygons, or pixel-wise segmentation. It is crucial for training models, especially in computer vision tasks like object detection and image segmentation.
2. Text Annotation
Text annotation includes named entity recognition (NER), sentiment analysis, and part-of-speech tagging. It is imperative for natural language processing (NLP) tasks and helps machines understand human language better.
3. Video Annotation
Video annotation is similar to image annotation but involves temporal data and movement. Labeling in this domain often requires marking objects/frame sequences, which is important in video surveillance and autonomous driving applications.
4. Audio Annotation
Audio annotation includes labelling sound clips with transcriptions, speaker identification, and sentiment analysis. This type of labelling aids in voice recognition and natural language processing tasks.
Implementing a Data Labelling Tool in Your Business
Integrating a data labelling tool into your business can be seamless if approached strategically. Here’s how:
Step 1: Assess Your Data Needs
Understand the types of data you handle and the volume of labelling required. This assessment helps in choosing the right tool and method.
Step 2: Choose the Right Tool
Select a data labelling tool that aligns with your organization's needs, budget, and technical expertise. Platforms like KeyLabs.ai are tailored to meet diverse observability, performance and cost requirements.
Step 3: Train Your Team
Ensure that your team receives adequate training on how to use the tool effectively. Even the best tools fail without proper usage and understanding of their features.
Step 4: Monitor and Evaluate
Continuously monitor the performance of your data labelling tool. Regular evaluations help in identifying any issues early on and adjusting processes or techniques as necessary.
KeyLabs.ai: A Pioneer in Data Labelling Tools
As a leading provider of data labelling tools, KeyLabs.ai stands out for its commitment to quality and innovation. With features that support various industries and applications, KeyLabs.ai caters to businesses that demand reliability and high performance in their data processes.
Some notable aspects that make KeyLabs.ai the preferred choice include:
- Scalability: The ability to handle both small-scale projects and large datasets effortlessly.
- Advanced AI Features: Utilizes cutting-edge AI techniques to enhance and automate the labelling process.
- Custom Solutions: Offers customizable solutions tailored to specific business needs.
- Robust Security: Ensures data privacy and security compliance, reassuring clients about their data safety.
Challenges in Data Labelling
While the use of data labelling tools offers numerous benefits, there are challenges that businesses need to address:
1. Data Privacy Concerns
Handling sensitive data requires strict adherence to data privacy regulations. Choosing a compliant data labelling tool is crucial for mitigating risks.
2. Quality Assurance
Maintaining consistency and accuracy across large datasets can be challenging. Implementing quality control processes within the labelling workflow can safeguard data integrity.
3. Evolving Requirements
As business needs change and evolve, so do the requirements for data labelling. Flexibility in the labelling tool’s functionalities can support changing demands and new data types.
Conclusion
The integration of a data labelling tool into your business process is not just an option; it’s a necessity for organizations aiming to leverage the full potential of AI and ML. Companies like KeyLabs.ai provide the infrastructure and expertise to enhance the data annotation process, ensuring that businesses have accurate and well-labelled datasets that can lead to informed decision-making and successful AI initiatives.
In summary, investing in a robust data labelling solution can enhance operational efficiency, reduce costs, and ultimately drive better business outcomes—a strategy essential for any modern organization competing in an increasingly data-driven world.
Explore KeyLabs.ai to find out how their data labelling tools can elevate your data capabilities and transform your AI initiatives.