Unlocking the Potential of Video Labeling Tools through Machine Learning
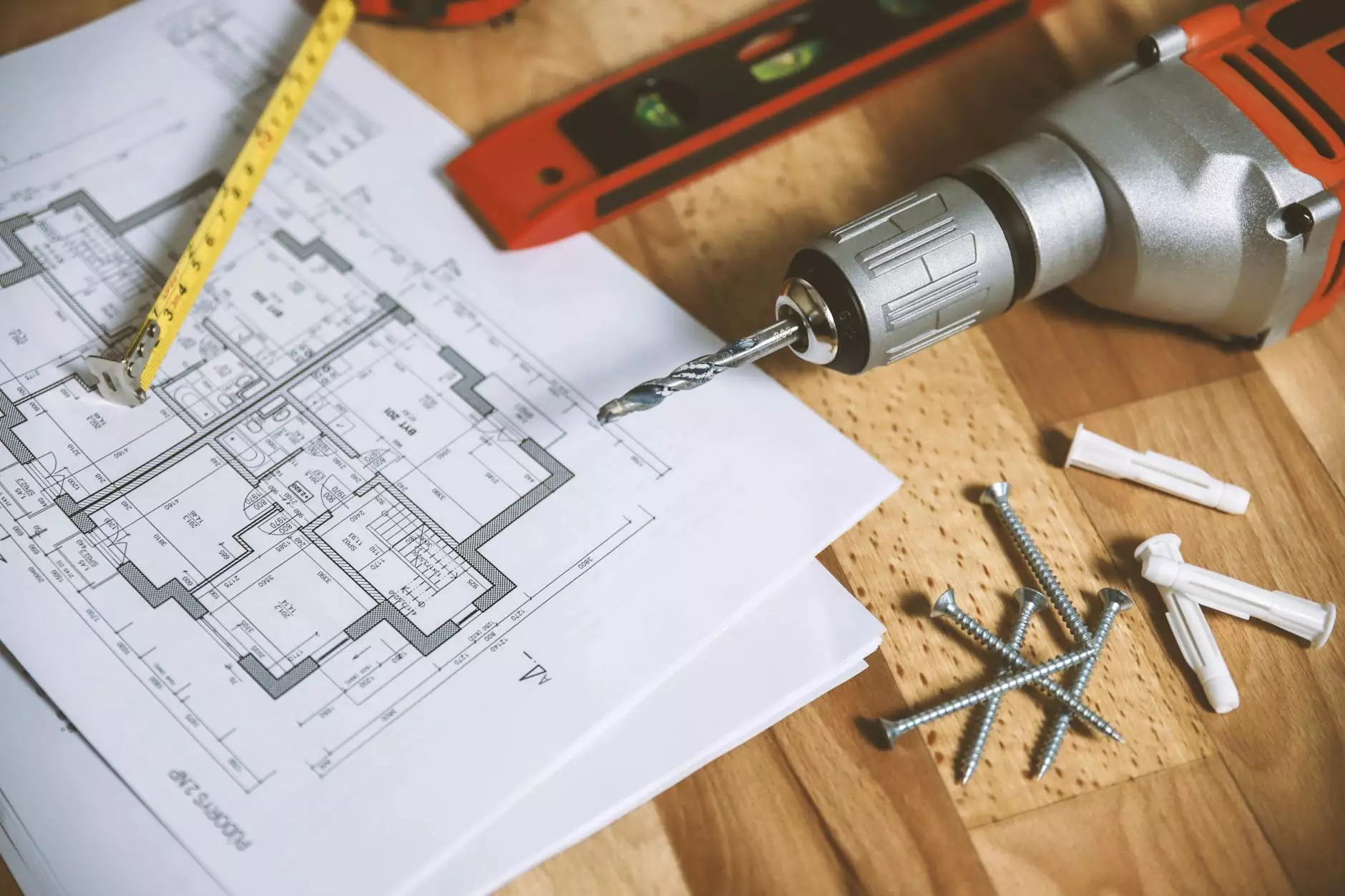
In today's data-driven world, the importance of effective data annotation cannot be overstated. With the advent of machine learning and AI technologies, businesses must embrace innovative solutions to keep pace with the ever-evolving landscape of data management. One such breakthrough is the video labeling tool powered by machine learning, which streamlines the annotation process, enhances accuracy, and ultimately drives better decision-making across various sectors.
Understanding Video Data Annotation
Video data annotation refers to the process of adding meaningful labels to video frames or segments. This task is crucial for training machine learning models, especially in computer vision applications. Properly annotated video data allows models to identify and understand objects, actions, and patterns within the footage.
Utilizing a video labeling tool equipped with machine learning capabilities can significantly enhance the efficiency of this process. Here are some key benefits:
- Increased Speed: Automated labeling dramatically reduces the time required for annotating large volumes of video footage.
- Improved Accuracy: Machine learning algorithms can assist in making precise annotations, minimizing human error.
- Scalability: Businesses can quickly scale their data annotation efforts to meet growing demands without compromising quality.
- Cost Efficiency: By automating the annotation process, companies can reduce labor costs associated with manual annotation.
The Role of Machine Learning in Video Labeling
Machine learning has revolutionized the way we approach video labeling. Traditional methods often require significant human input, which can lead to inconsistencies and errors. However, machine learning algorithms can analyze large datasets and learn from patterns to automate this task effectively.
Types of Video Labeling Tools
There are several types of video labeling tools that utilize machine learning, each designed to meet specific business needs. Here are a few notable examples:
- Object Detection Tools: These tools focus on identifying and labeling specific objects within video frames, useful in surveillance and security applications.
- Action Recognition Platforms: They can analyze video sequences to detect and annotate actions, ideal for sports analytics and behavioral studies.
- Facial Recognition Software: This type of tool uses machine learning to recognize and label faces in videos, applicable in the security and marketing sectors.
Implementing a Video Labeling Tool: A Step-by-Step Guide
Implementing a video labeling tool machine learning approach involves several steps. Below is a detailed guide on how to effectively integrate these tools into your data annotation workflow:
1. Define Your Objectives
Before starting, clearly define what you aim to achieve with video annotation. Are you interested in object detection, action recognition, or sentiment analysis? Understanding your goals will guide you in selecting the right tools and techniques.
2. Choose the Right Tool
Research various video labeling tools available in the market. Look for features that align with your project requirements, such as:
- User-friendliness
- Support for various video formats
- Integration capabilities with existing systems
- Robust machine learning algorithms
3. Data Preparation
Prepare your video data by organizing and preprocessing it for labeling. This can include:
- Segmenting longer videos into manageable clips
- Ensuring high-quality video resolution for better accuracy
- Providing context for the annotators regarding the objectives of the labeling task
4. Training the Model
Utilize a video labeling tool machine learning framework to train your model on the prepared dataset. Machine learning algorithms will analyze the annotated data and learn to make predictions on new, unlabelled videos.
5. Annotate and Evaluate
Start annotating the videos using your selected tool. Continuously evaluate the performance of the machine learning model and make necessary adjustments to improve accuracy.
6. Quality Control
Implement a quality control process to ensure that the annotations meet your standards. This can involve a combination of automated checks and manual reviews by human annotators.
7. Deployment and Feedback Loop
Once satisfied with the results, deploy the model in your production environment. Establish a feedback loop to continually improve the model based on real-world performance data.
Applications of Video Labeling Tools
The versatility of video labeling tools driven by machine learning allows them to be applied across various industries:
1. Automotive Industry
In the automotive sector, video labeling tools are used to develop and enhance advanced driver-assistance systems (ADAS). By annotating videos captured from vehicle cameras, machine learning models can be trained to recognize traffic signs, pedestrians, and other vehicles, contributing to safer driving experiences.
2. Security and Surveillance
Security agencies use video labeling to monitor CCTV footage for suspicious activities. By implementing automated labeling tools, organizations can quickly review hours of footage to identify potential threats and respond promptly.
3. Entertainment and Sports
In sports, video labeling technology helps analysts break down plays, assess player performance, and improve strategic decisions. By labeling and analyzing game footage, teams can gain insights into gameplay patterns and opponent strategies.
4. Healthcare
In healthcare, video labeling can assist in monitoring patient behavior or analyzing movement patterns in physical therapy sessions. By annotating these videos, healthcare professionals can tailor treatment plans to individual patient needs.
Challenges and Solutions in Video Labeling
Although the benefits of using video labeling tools are substantial, there are inherent challenges associated with the process. Understanding these obstacles and addressing them is essential for successful implementation.
1. Data Privacy Concerns
As videos often contain sensitive information, maintaining data privacy is crucial. Companies should ensure compliance with regulations such as GDPR and HIPAA when handling video data.
2. High Initial Setup Costs
Effective video labeling tools can be expensive to implement. However, the return on investment can be significant when considering long-term productivity gains and efficiency improvements.
3. Quality of Annotated Data
Ensuring the quality of annotated data is essential for building accurate machine learning models. Continuous training, quality assurance processes, and human oversight can mitigate this issue.
The Future of Video Labeling with Machine Learning
The future of video labeling tools looks promising as machine learning continues to advance. Innovations in deep learning and neural networks will likely lead to even more sophisticated annotation capabilities, allowing for real-time video analysis and improved model accuracy.
As industries increasingly rely on video data for insights, adopting these tools will become a competitive necessity. Companies that harness the power of video labeling tools machine learning will not only enhance their data annotation processes but also gain a strategic advantage in the marketplace.
Conclusion
In conclusion, leveraging video labeling tools embedded with machine learning capabilities is crucial for organizations aiming to stay competitive in an data-centric era. The uniqueness of these tools lies in their ability to automate complex tasks, ensuring efficient and precise data annotation. By recognizing the diverse applications and addressing challenges, businesses can effectively incorporate these tools, leading to enhanced operational efficiency and better decision-making capabilities.
By understanding the intricacies of video labeling and implementing effective strategies, you can unlock the full potential of your data, drive innovation, and facilitate a data-informed culture within your organization. As the demand for annotated video content continues to grow, platforms like KeyLabs.ai are well-positioned to provide cutting-edge solutions that meet the needs of various industries.